Important Keyword: Hypothesis Testing, Null Hypothesis, Alternative Hypothesis, Statistical Significance.
Words: 2691, Read Time: 14 Min
Table of Contents
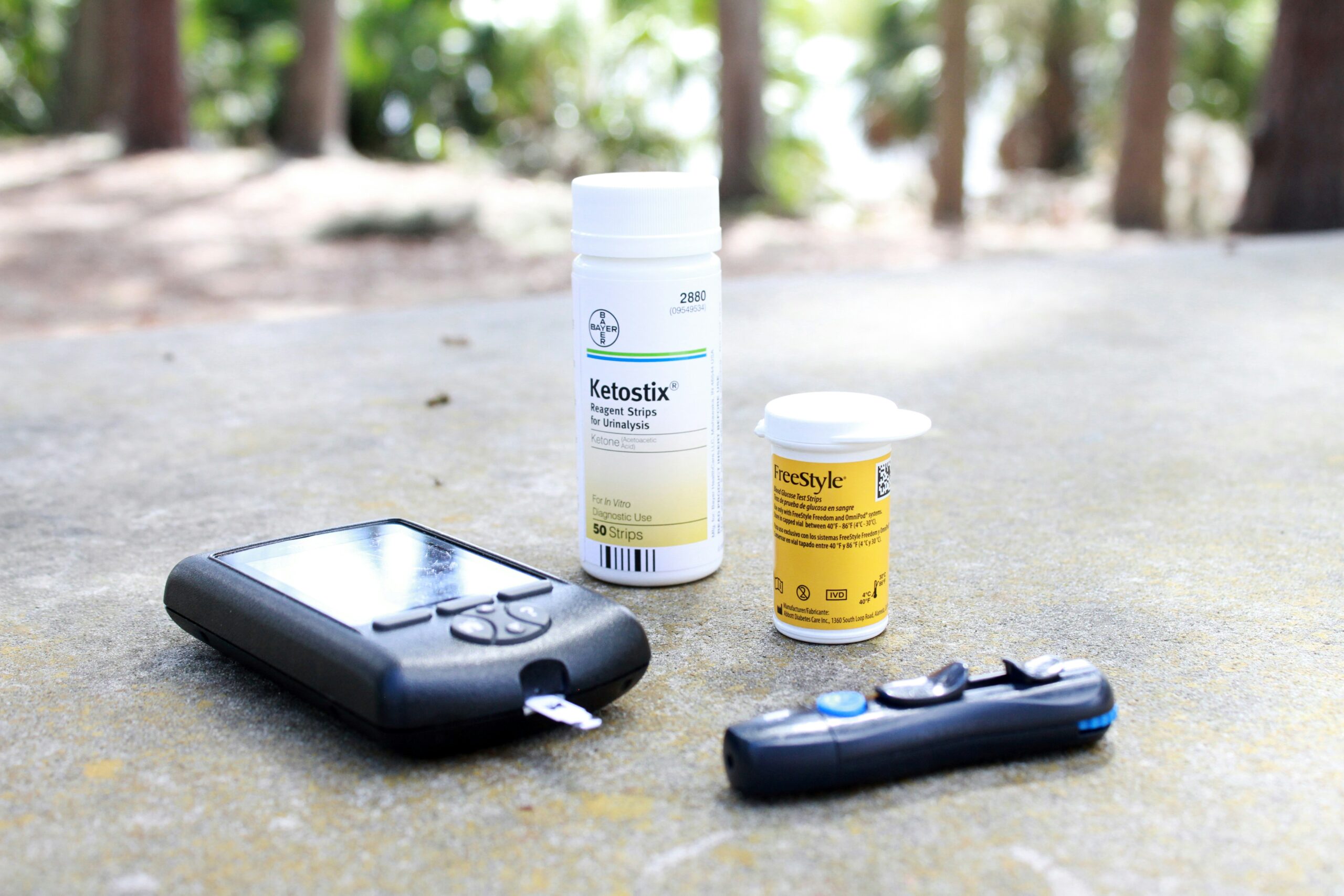
Introduction to Hypothesis Testing
Hypothesis testing is a pivotal aspect of statistical analysis, providing a structured framework for making decisions based on sample data. At its core, hypothesis testing involves formulating a specific assumption or conjecture about a population parameter—such as a mean or proportion—and using statistical methods to determine the validity of that assumption. This process is integral for researchers and analysts in fields ranging from medicine to social sciences, as it allows them to draw conclusions about broader groups based on limited observations.
The significance of hypothesis testing lies in its ability to gauge the likelihood that a particular statement about a population holds true. When a hypothesis is tested, analysts typically define two opposing statements: the null hypothesis, which posits that there is no effect or difference, and the alternative hypothesis, which suggests the contrary. Through various statistical techniques, researchers assess the evidence against the null hypothesis, thus informing their understanding of the phenomenon under observation.
Moreover, hypothesis testing serves as a tool for risk management in decision-making processes. By quantifying the probability of observing sample results under the assumption that the null hypothesis is true, analysts can establish a clear threshold for determining statistical significance. This enables them to make informed recommendations while accommodating uncertainty and variability present in real-world data.
As one delves deeper into hypothesis testing, it becomes clear that this technique does not merely apply to academic research; it is immensely valuable in business, healthcare, and public policy. By rigorously analyzing data and evaluating hypotheses, analysts contribute to evidence-based practices that enhance decision-making and optimize outcomes. Understanding the components and implications of hypothesis testing is essential for anyone looking to leverage statistical analysis effectively.
What is a Hypothesis?
A hypothesis is a foundational concept in the field of statistics, serving as a statement that can be tested through empirical investigation. It is essentially an educated guess about the relationships between variables or the characteristics of a population. In the context of hypothesis testing, two types of hypotheses are particularly important: the null hypothesis (often symbolized as H0) and the alternative hypothesis (denoted as Ha or H1).
The null hypothesis is a statement that assumes no effect or no difference exists in the population being studied. It posits that any observed effects in the sample data are purely due to random chance. Conversely, the alternative hypothesis challenges this assumption, suggesting that there is a statistical significance or a real effect present in the data. For instance, if a researcher is analyzing the impact of a new drug, the null hypothesis would assert that the drug has no effect on patients, while the alternative hypothesis would claim that it does.
One of the critical aspects of these hypotheses is their mutual exclusivity; they cannot be true at the same time. At the conclusion of the hypothesis testing process, based on the collected data, one hypothesis will either be rejected or not rejected. This binary decision-making process is central to validating or refuting the assumptions made about the population or phenomenon in question.
Additionally, it is important to note that rejecting the null hypothesis does not definitively prove the alternative hypothesis; it merely suggests that the data provides sufficient evidence against the null. This framework is pivotal in various scientific disciplines, allowing researchers to make informed decisions based on statistical analysis.
The Four Steps of Hypothesis Testing
Hypothesis testing is a fundamental process in statistical analysis, aimed at making inferences about populations based on sample data. The procedure generally involves four essential steps: stating the hypotheses, creating an analysis plan, executing the analysis, and analyzing the results. Each of these steps plays a crucial role in ensuring a robust testing methodology.
The first step is to clearly state the hypotheses. This typically involves formulating a null hypothesis (H0), which represents the status quo or a claim of no effect, and an alternative hypothesis (H1), which signifies the presence of an effect or a difference. These hypotheses need to be specific and measurable, as they guide the entire testing process.
Once the hypotheses are established, the second step entails creating an analysis plan. This involves selecting the appropriate statistical tests based on the type of data and the hypotheses formed. Factors such as the sample size, distribution characteristics, and the significance level (alpha) must be defined. This planning stage is crucial, as it lays the groundwork for how the analysis will be executed.
The third step is executing the analysis. In this phase, data collection and measurement need to take place according to the analysis plan developed in the previous step. It involves performing the statistical tests and computing the relevant statistics such as p-values or confidence intervals, which will inform the conclusions drawn from the test.
Finally, the fourth step involves analyzing the results. This includes interpreting the findings in the context of the original hypotheses. It is essential to determine whether the null hypothesis can be rejected or not, based on the p-value and the predefined significance level. This step also includes discussing the practical significance and implications of the results, ensuring that conclusions drawn from the analysis are relevant to the hypothesis testing process. Each of these steps is interconnected, forming a cohesive framework essential for effective hypothesis testing.
Importance of Hypothesis Testing
Hypothesis testing holds a crucial place in statistical analysis as it provides a systematic method for evaluating claims about populations based on sample data. It allows researchers to ascertain whether the evidence collected supports or refutes their initial assumptions, termed hypotheses. This method of testing is instrumental across various disciplines, including sociology, psychology, medicine, and business, making it a vital tool for data-driven decision-making.
In essence, hypothesis testing facilitates the assessment of the credibility of a claim. When researchers formulate a hypothesis, they often outline a null hypothesis (H0), which represents no effect or relationship, and an alternative hypothesis (H1), indicating the presence of an effect or relationship. Through statistical procedures, researchers can determine the probability of observing their data assuming the null hypothesis is true. If this probability, denoted by the p-value, falls below a predetermined significance level, the null hypothesis is rejected, leading to the conclusion that the findings are statistically significant.
The implications of hypothesis testing extend beyond mere acceptance or rejection of a hypothesis. In fields like medicine, for instance, it plays a fundamental role in validating the effectiveness of treatments or the safety of new drugs. In social sciences, it aids in understanding behavioral patterns and societal trends. Similarly, businesses utilize hypothesis testing to analyze consumer preferences, predict market trends, and enhance operational efficiency. Consequently, the practice promotes empirical evidence over assumptions, contributing greatly to informed decision-making and policy formation.
Overall, the ability to test hypotheses rigorously underscores its importance in scientific exploration and data analysis. By establishing a framework for evaluating evidence, hypothesis testing ensures that conclusions drawn are based on statistical significance, thus boosting confidence in the findings across various fields.
Advantages and Disadvantages of Hypothesis Testing
Hypothesis testing is a fundamental statistical approach that offers various advantages, contributing significantly to data interpretation and decision-making processes. One of the primary benefits of hypothesis testing is its ability to provide a structured framework for evaluating data. By formulating a null hypothesis and an alternative hypothesis, researchers can objectively assess the evidence against the null, leading to informed conclusions. This structured approach minimizes subjective bias and enhances the credibility of research findings.
Another advantage is the clarity it provides in interpreting results. The use of p-values and confidence intervals allows researchers to quantify the strength of evidence supporting or refuting a hypothesis, making the subsequent interpretation clearer and more systematic. This quantitative assessment aids in determining the practical significance of findings, thus enhancing the applicability of statistical results in real-world scenarios.
However, despite its advantages, hypothesis testing is not without drawbacks. One critical disadvantage is the potential for misinterpretation of results. A common issue arises from the reliance on p-values, which can lead to erroneous conclusions if not properly understood. For example, a p-value below the chosen significance level is often mistakenly interpreted as strong evidence for an alternative hypothesis, disregarding the possibility of Type I errors.
Furthermore, hypothesis testing often relies on certain assumptions regarding the dataset, such as normality and independence. When these assumptions are violated, the results may become invalid or misleading, leading researchers to draw incorrect conclusions. This reliance on potentially flawed assumptions highlights the need for cautious application of hypothesis testing, as it may not always capture the complexities of real-world data accurately.
In conclusion, while hypothesis testing offers critical advantages in statistical analysis, including structured decision-making and clarity in data interpretation, it is essential to remain vigilant regarding its limitations. Understanding both the benefits and drawbacks is crucial for effectively employing hypothesis testing in various research contexts.
Example of Hypothesis Testing in the Indian Context
Hypothesis testing is a crucial statistical method employed across various fields, including social sciences, healthcare, and business. To illustrate its application in the Indian context, let us consider the example of a government initiative aimed at improving the nutritional status of children in rural India. The government has introduced a new fortification program aimed at enhancing the iron levels in the diets of these children.
Initially, the health department sets a null hypothesis (H0) stating that the fortification program has no effect on the iron levels in children’s blood. Conversely, the alternative hypothesis (H1) posits that the program does have a positive effect on enhancing iron levels. To test these hypotheses, researchers would collect blood samples from a representative group of children before and after the implementation of the fortification initiative.
Once the data is gathered, statistical analysis will be conducted to evaluate any significant differences in the iron levels pre- and post-intervention. For instance, using a significance level of 0.05, if the p-value obtained from the analysis is less than this threshold, it indicates sufficient evidence to reject the null hypothesis in favor of the alternative hypothesis, thus confirming the effectiveness of the program.
This practical approach to hypothesis testing not only highlights the effectiveness of the nutritional program but also demonstrates its vital role in public health initiatives. By applying this method rigorously, policymakers can make informed decisions that ultimately contribute to the improvement of children’s health in India. Such a scenario reflects the broader importance of hypothesis testing as a tool for assessing the outcomes of various interventions, thereby guiding future actions based on data-driven results.
Frequently Asked Questions about Hypothesis Testing
Hypothesis testing can often raise various questions for beginners. Understanding these inquiries is crucial for grasping the overall concept and application of statistical testing.
One common question is: “What is the difference between type I and type II errors?” A type I error occurs when one rejects a true null hypothesis, essentially saying that there is an effect or difference when none actually exists. Conversely, a type II error is the failure to reject a false null hypothesis, which means one concludes that there is no effect or difference when there actually is one. These errors help define the potential pitfalls of hypothesis testing and are vital in determining the accuracy of statistical findings.
Another frequently asked question is: “When should I use hypothesis testing?” Hypothesis testing is applicable when making decisions or inferences about population parameters based on sample data. It is particularly useful when dealing with uncertainties in data, allowing researchers to make statistically sound conclusions. Key situations for employing hypothesis testing include evaluating the effectiveness of new treatments, comparing group means, and analyzing relationships between variables in research. Thus, it serves as an essential tool for researchers and professionals when trying to draw insights from data.
Individuals may also wonder about the significance level, often denoted as alpha (α). This threshold determines the probability of committing a type I error and is a critical component in hypothesis testing. Common significance levels, such as 0.05 or 0.01, indicate the likelihood of rejecting a true null hypothesis. Lastly, understanding p-values, which indicate the strength of evidence against the null hypothesis, is crucial to interpreting results accurately. A small p-value suggests significant evidence that the null hypothesis should be rejected.
Key Takeaways
Hypothesis testing is a foundational concept in statistics that plays a crucial role in decision-making across various fields. One of the primary insights gleaned from our discussion is that hypothesis testing allows researchers and analysts to make informed inferences about population parameters based on sample data. This method relies on constructing two competing hypotheses: the null hypothesis, which posits no effect or difference, and the alternative hypothesis, which suggests a variation exists.
Another important aspect of hypothesis testing is the significance level, commonly denoted as alpha (α). This threshold determines the probability of rejecting the null hypothesis when it is true. By convention, a significance level of 0.05 is widely used, indicating a 5% risk of concluding that a difference exists when there is none. Understanding the implications of this value is essential for interpreting test results and managing the risk of Type I errors, where a true null hypothesis is incorrectly rejected.
Moreover, the concept of p-values serves as a pivotal component in hypothesis testing. The p-value measures the strength of evidence against the null hypothesis. A smaller p-value suggests stronger evidence that supports the alternative hypothesis. Consequently, the relationship between p-values and the significance level is critical, as it aids in making determinations about hypotheses drawn from experimental or observational data.
Finally, it is vital to acknowledge the practical applications of hypothesis testing in diverse fields, including healthcare, psychology, and social sciences. The ability to validate research findings or evaluate the effectiveness of interventions relies heavily on robust hypothesis testing methodologies. Understanding these fundamental concepts equips individuals with the necessary tools to critically evaluate research outcomes and draw valid conclusions from empirical studies.
Conclusion
In conclusion, hypothesis testing stands as a pivotal framework in the realm of statistics and research methodologies. Its core purpose is to assist researchers in making data-driven decisions by evaluating the validity of assumptions regarding a certain population parameter. Throughout this guide, we have explored the fundamental concepts that underpin hypothesis testing, including null and alternative hypotheses, significance levels, p-values, and types of errors. These elements work in concert to provide a structured approach to analyzing relevant data.
Moreover, the role of hypothesis testing extends beyond academia into various fields, such as business, healthcare, and social sciences. By applying hypothesis testing techniques, professionals can draw meaningful conclusions from data, improve decision-making processes, and ultimately enhance operational efficiency. In today’s data-centric world, where informed choices are paramount, understanding hypothesis testing has become more relevant than ever.
Moreover, the application of hypothesis testing teaches us to embrace uncertainty and recognize the probabilistic nature of conclusions drawn from empirical data. It refines our analytical skills, enabling us to discern valid inferences from mere coincidence, fostering a culture of evidence-based decision-making. As we navigate through a landscape increasingly influenced by data analysis, the knowledge of hypothesis testing equips us with the tools necessary to make sense of complex information and derive actionable insights.
As you incorporate these techniques into your own analyses, remember that the true value of hypothesis testing lies not only in its ability to confirm or reject hypotheses but also in its capacity to encourage critical thinking and continuous learning. Therefore, we encourage you to delve deeper into this vital topic and explore its numerous applications to enhance your analytical prowess.
Download Pdf: https://taxinformation.cbic.gov.in/